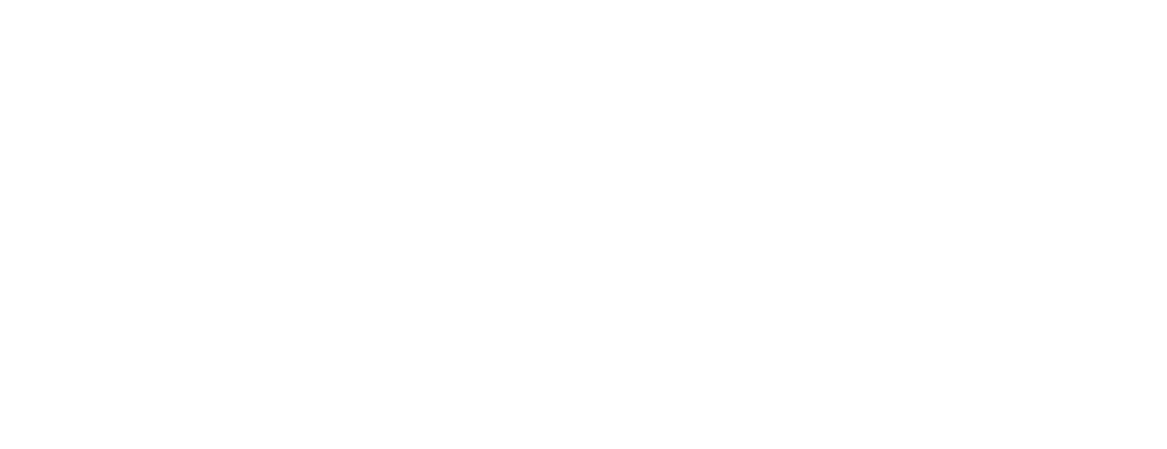
Bring your data to live!
GreyCat is a programmable temporal graph database, made for efficient and flexible data processing at scale
Database system
Efficient storing and retrieval of relational, temporal and geographic data as a graph.
Object-Oriented Programming
Break free from the query chains and enjoy the flexibility of object-oriented data processing.
Data Analytics Stack
Integrated tools and libraries to identify and visualize crucial insights of your data
Install GreyCat for free
Documentation InstallOur community version is completely free and should suffice to start building your project without any resource limitation
A Programable Database
All the benefits of a graph database without needing to learn a new fringe syntax.
No Query
No need for complex queries, traverse a graph as you would a simple object with dot notation.
No Mapping
A single Data Model from Disk to Api.
Any Scale
Datasets do not impose the infrastructure anymore, Hardware defines the speed.
No Tabular
No complex join or filters, leverage Object-Oriented traversal of the graph.
What If, Many Worlds
Simulate and store branches of your current state in a simple manner.
Stateful programming
Reduce the cost and time of processing by keeping the state and resume from where you left on the next iteration.
Script or serve
Use GreyCat as a stateful scripting solution or serve webapps (backend & frontend) from one executable.
How Does It Work ?
From input to insight in 3 simple steps
Example Data Source
This dataset represents sensor readings taken at various global locations, with each row capturing the following information:
- Latitude and Longitude: Coordinates of the sensor location
- Sensor ID: Unique identifier for each sensor
- Timestamp: Unix timestamp representing the date and time of the reading
- Humidity: Humidity levels in percentage
- Temperature: Temperature values in degrees Celsius
latitude,longitude,sensor_id,timestamp,humidity,temperature
40.712776,-74.005974,S001,1694508300,65.2,22.5
34.052235,-118.243683,S002,1694508360,58.7,24.0
51.507351,-0.127758,S003,1694508420,72.1,18.3
48.856613,2.352222,S004,1694508480,69.0,19.6
35.689487,139.691711,S005,1694508540,55.5,23.8
55.755825,37.617298,S006,1694508600,60.4,16.9
-33.868820,151.209290,S007,1694508660,64.8,20.1
19.432608,-99.133209,S008,1694508720,62.3,21.7
52.520008,13.404954,S009,1694508780,68.9,17.4
-23.550520,-46.633308,S010,1694508840,59.1,25.3
type Sensor {
id: String;
values: nodeTime<Measurement>;
}
type Measurement {
temperature: float;
humidity: float;
}
// global variable, serve as graph entry-point
var sensor_index: nodeGeo<Sensor>;
Step 1: Data Modeling
- Sensor: This object holds the id of each sensor and a list of Measurement values associated with it.
- Measurement: Contains two attributes, temperature and humidity, represented as floating-point values.
- A global variable, sensor_index, is used as the entry point of the data graph. It organizes sensors by geographical location.
This structured data model enables efficient organization and retrieval of sensor data for further analysis and operations.
Step 2: Import
- The
import()
function reads the CSV file located at "data.csv" using aCsvReader
object. -
In the
CsvFormat
object we only need to specify which column represents time, to automatically read the values as our internal nativetime
type. - For each line of data, the code extracts geographical coordinates (latitude and longitude) and looks up the corresponding sensor in the sensor_index.
- If the sensor doesn't exist, it creates a new
Sensor
object and associates it with the given location. - A new
Measurement
object is created from the CSV values for humidity and temperature, and it's stored in the sensor's values at the correct timestamp.
fn import() {
var format = CsvFormat { columns: [CsvColumnTime { offset: 3 }] };
var cr = CsvReader::new("data.csv", format);
while (cr.available() > 0) {
var line = cr.read();
var geoIndex = geo::new(line[0], line[1]);
var sensor = sensor_index.resolve(geoIndex);
if (sensor == null) {
sensor = Sensor {
id: line[2],
values: nodeTime<Measurement>::new()
};
sensor_index.set(geoIndex, sensor);
}
var measurement = Measurement {
humidity: line[4],
temperature: line[5]
};
sensor.values.setAt(line[3] as time, measurement);
}
}
@expose
fn temperature_std(center: geo, radius: float, t: time): float {
var gaussian = Gaussian {};
var circle = GeoCircle::new(center, radius);
for (sensor_location, sensor in sensor_index) {
if (circle.contains(sensor_location)) {
gaussian.add(sensor.values.resolveAt(t)?.temperature);
}
}
return gaussian.std();
}
Step 3: Spatial + Temporal Analysis
The temperature_std()
function calculates the standard deviation of temperature
readings within a specified geographic area and time:
- The
@expose
keyword exposes the function as callable API over HTTP protocols - The function takes three parameters: a center location, a radius, and
a
time
t. - A
GeoCircle
object is created to represent the defined geographic area. - For each sensor in the sensor_index, if its location falls within the circle, the
temperature at the given time is added to a
Gaussian
object; representing the distribution. - Finally, the standard deviation of the temperatures within the circle at the given time, is returned.
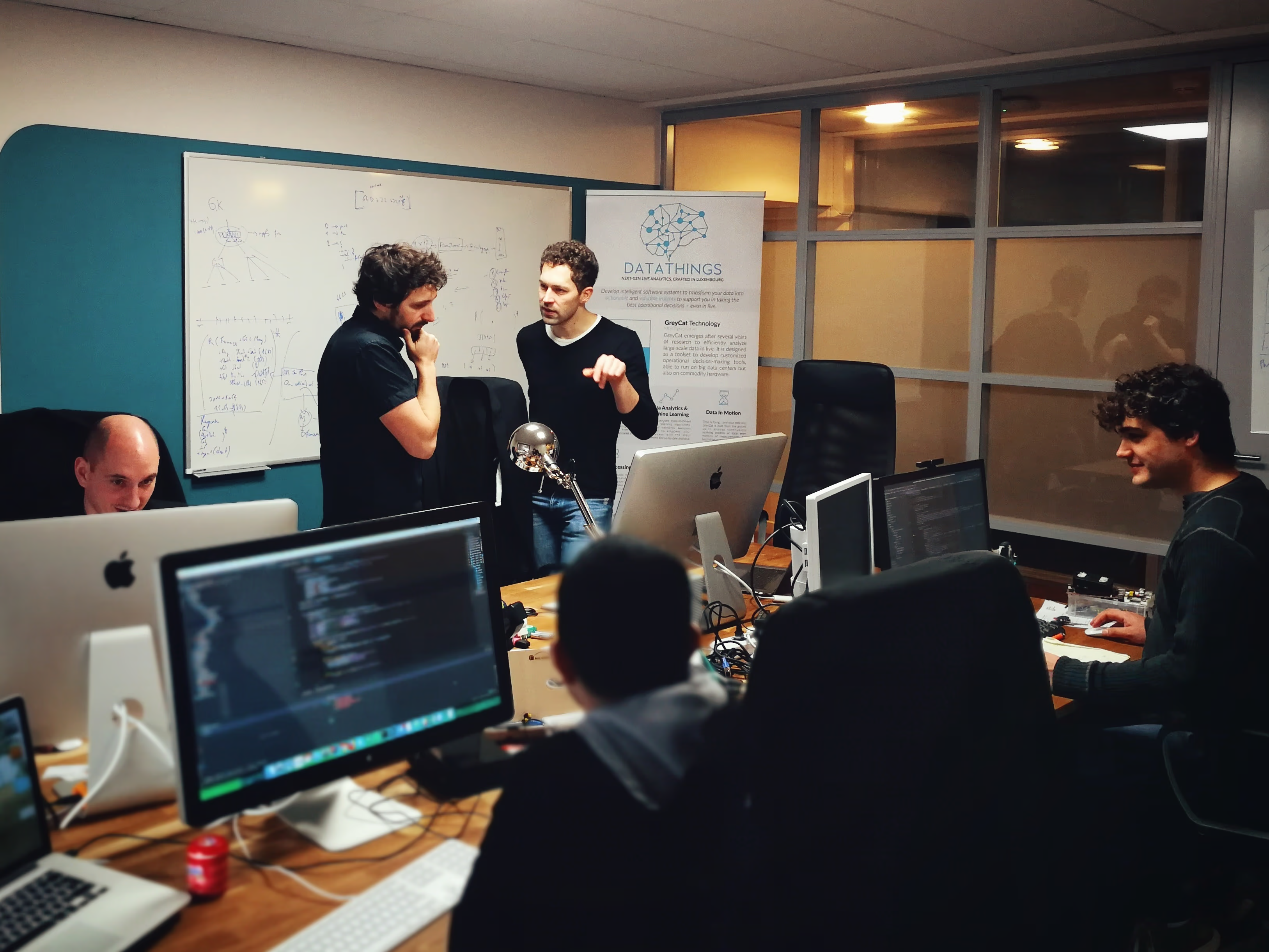
The history of GreyCat
From a theoretical idea into a fully fledged programming language managing & simulating an entire Countries energy grid
Learn moreWant to learn more about GreyCat ?
Our Blog Posts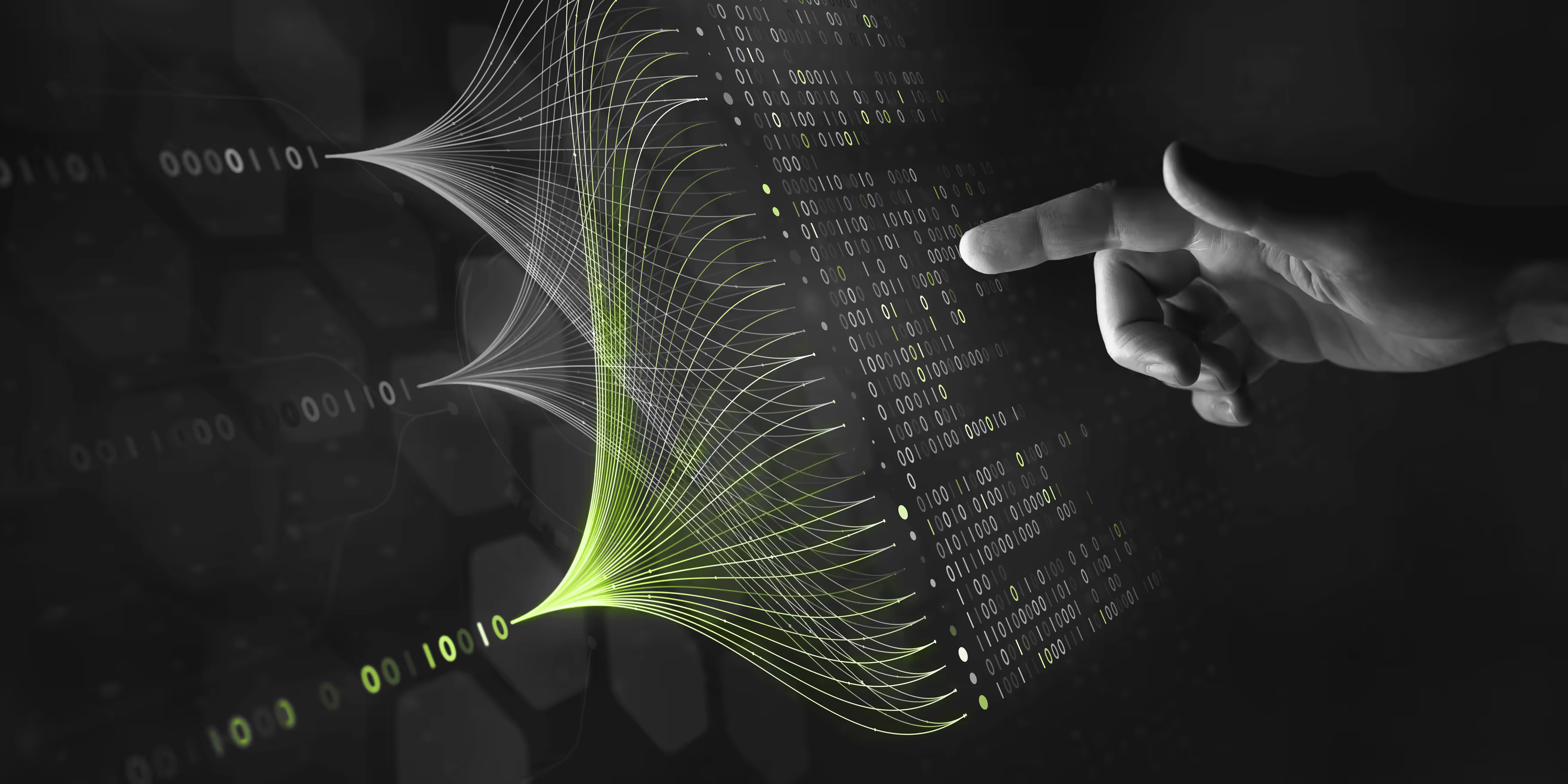